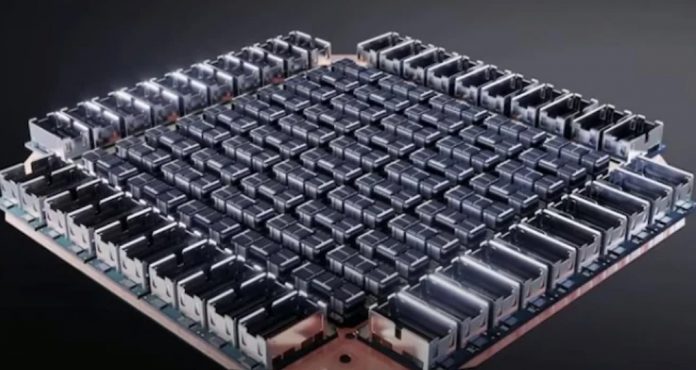
In the ever-intensifying global race for artificial intelligence supremacy, Tesla has made a bold and strategic move that could redefine its future—not just as an electric vehicle manufacturer, but as a frontrunner in AI infrastructure. At the heart of this transformation lies Dojo, Tesla’s custom-built supercomputer designed to accelerate AI training, enhance Full Self-Driving (FSD) capabilities, and power the next wave of intelligent machines like the Optimus humanoid robot.
Unlike most AI companies that depend on third-party GPU suppliers, Tesla is carving its own path with Dojo, a gamble that CEO Elon Musk admits is high-risk, but potentially game-changing. While Nvidia’s dominance in the GPU market continues to soar, Tesla’s push to build its own AI supercomputing backbone underscores a broader ambition: technological independence, economic scalability, and global AI leadership.
This article dives deep into the origins, purpose, architecture, and future implications of the Dojo supercomputer—and why this might be Tesla’s most pivotal innovation yet.
The Origins of Dojo: From Concept to Crucial Infrastructure
When Elon Musk first teased the concept of a Tesla-built supercomputer at the company’s inaugural Autonomy Day in 2019, the idea seemed audacious. At the time, GPUs were largely confined to gaming and rendering tasks, and supercomputers were typically reserved for niche scientific applications like climate modeling or genome sequencing.
However, in just five years, the technological landscape has shifted dramatically. The explosion of generative AI and machine learning has made powerful GPU clusters indispensable. As the world clamors for more AI compute, Tesla’s early bet on Dojo now appears prescient.
Why Tesla Needs Dojo: Scarcity, Cost, and Strategic Control
Tesla has long relied on Nvidia’s GPU hardware for training its neural networks, investing billions in the company’s H100 and H200 chips. In fact, Tesla’s recent Cortex data center at Giga Texas houses approximately 50,000 Nvidia H100 GPUs. Simultaneously, Elon Musk’s xAI startup is constructing an even larger AI training facility in Memphis, Tennessee, which is expected to house up to 100,000 Nvidia chips—underscoring just how essential these processors are to Musk’s AI ambitions.
But this reliance poses a significant vulnerability. As Musk has noted, the demand for Nvidia chips is so intense that even deep-pocketed firms like Tesla face supply bottlenecks. Musk’s response is pragmatic: the best supplier is no supplier. By building Dojo in-house, Tesla aims to ensure uninterrupted access to AI compute while simultaneously reducing long-term dependency and costs.
Economically, the logic is compelling. While the initial investment for Dojo—reportedly around $500 million for version 1—is steep, it could significantly reduce the cost per unit of AI training over time. Musk has floated the idea that Dojo could eventually become a revenue-generating platform akin to Amazon Web Services (AWS), offering AI compute as a service to other companies.
What Is Dojo? A Purpose-Built AI Training System
So, what exactly is Dojo? Unlike generic supercomputers built from off-the-shelf parts, Dojo is purpose-engineered by Tesla for AI model training—specifically the computer vision systems that power Tesla’s FSD and robotics programs.
The heart of Dojo is the D1 chip, a custom Tesla-designed processor built at TSMC in Taiwan. Unlike Nvidia GPUs, which are general-purpose and highly flexible, the D1 chip and its associated architecture are specialized for one thing: high-throughput AI training. This specificity means Tesla can potentially achieve far greater efficiency for its own workloads than it could with Nvidia’s more universal chips.
Dojo is currently housed in a data center in Buffalo, New York—a location chosen perhaps for its cold climate (ideal for cooling), stable infrastructure, and access to green energy via hydroelectric dams. While version 1 of Dojo is modest in raw scale—estimated to deliver performance equivalent to 8,000 Nvidia H100 GPUs—it is highly optimized for Tesla’s unique needs.
The Dual Path Strategy: Nvidia + Dojo
Tesla isn’t putting all its eggs in one basket. The company is pursuing a dual-path AI infrastructure strategy: continue to use Nvidia GPUs where it makes sense while building out Dojo as a parallel and eventually dominant system. This balanced approach allows Tesla to remain competitive in the short term while investing in a more scalable and self-reliant future.
Musk has also confirmed that Dojo V1 is already online and performing productive AI training tasks. The roadmap includes plans for future versions—Dojo 1.5, Dojo 2, Dojo 3—which will presumably increase in scale, flexibility, and application range.
Despite the excitement, Musk has been transparent about the risks. Dojo is a long-shot bet, but one with a high potential reward. If successful, it could allow Tesla not only to train AI models faster and cheaper but also to evolve into a full-fledged AI platform company.
Applications: From Full Self-Driving to Humanoid Robots
The first and most obvious application for Dojo is Tesla’s Full Self-Driving system. Achieving true autonomy requires processing and labeling immense volumes of video data—something that Dojo is custom-built to accelerate.
With the robotaxi vehicle slated for unveiling in October 2025, the pressure is on. This vehicle will be judged entirely on its software capabilities, meaning the AI must be flawless. Dojo’s ability to rapidly iterate and refine these models will be crucial.
But FSD is just the beginning. Tesla also plans to use Dojo to train AI for its humanoid robot, Optimus. Right now, the training is limited to vision and navigation tasks, much like FSD. But Musk envisions a future where Optimus robots are as ubiquitous as smartphones, helping with household chores, elderly care, and even industrial labor. To support this, Dojo will eventually need to train more generalized AI models, moving beyond vision to include language, reasoning, and manipulation tasks.
This expansion would require a new generation of Dojo hardware—a transition Musk hinted at when he said Dojo V2 will “address current limitations.”
Scaling Global AI: Dojo and the Road Ahead
One of the most compelling justifications for Dojo is the need to scale AI across geographies. While Tesla has amassed an enormous dataset from U.S. and Chinese roads, global deployment of FSD will require equally comprehensive data from every corner of the world—Brazil, India, Europe, Southeast Asia.
Every new environment introduces new road signs, languages, driving behaviors, and infrastructure quirks. Training AI for these diverse conditions will require exponentially more data—and compute power. Dojo can make that training not only feasible but cost-effective.
Tesla’s long-term vision appears to be the creation of a vertically integrated AI empire: data collection from its vehicle fleet, AI training on Dojo, inference on Tesla-designed chips inside vehicles, and real-world deployment in both cars and robots. It’s a loop that no other automaker—or tech company—currently controls end to end.
Economic Outlook: Cost vs. Capability
From a financial standpoint, Dojo’s costs are significant but strategic. Elon Musk has revealed that Tesla’s AI expenditures in 2024 will reach around $10 billion. Roughly half of that will be internal R&D, including the vehicle inference chips and Dojo supercomputing clusters. Nvidia hardware will still account for $3–4 billion—about two-thirds of the hardware spend.
Despite being a smaller slice of the pie, Dojo offers something Nvidia can’t: long-term cost savings and customizability. If Tesla can match or surpass Nvidia’s performance with a system optimized for its own tasks, the economics could tilt decisively in Dojo’s favor.
Moreover, by owning the hardware and the software stack, Tesla opens the door to entirely new business models—whether it’s selling Dojo-as-a-Service to other AI firms, licensing the architecture, or offering hosted training for third-party autonomous systems.
Final Thoughts: A Calculated Gamble That May Just Pay Off
Tesla’s Dojo project exemplifies the kind of calculated risk that defines innovation. It’s a multimillion-dollar moonshot aimed at securing Tesla’s leadership in both automotive and robotics AI. The stakes are high, the road is uncertain, and the returns are anything but guaranteed.
Yet, in an industry where access to compute is fast becoming the most valuable resource, Tesla’s decision to build rather than buy could set it apart. Dojo isn’t just a supercomputer—it’s a strategic fulcrum around which Tesla is balancing its future.
If Musk and his team succeed, Dojo could become the cornerstone of not only Tesla’s next-gen vehicles and humanoid robots but the global AI economy itself.