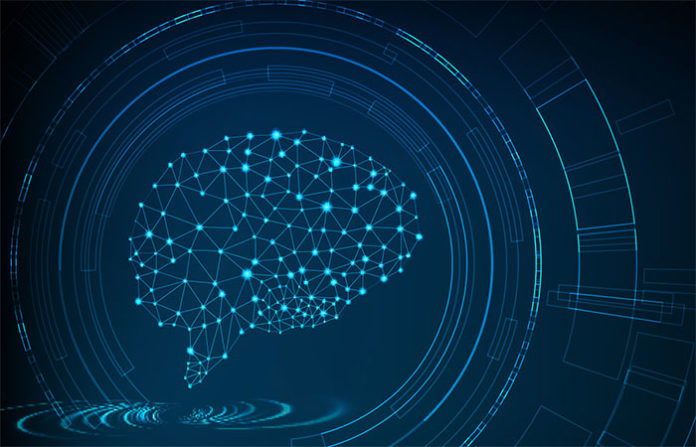
Intelligent automation (IA) is revolutionizing how tasks are allocated and performed within enterprises. Initially introduced in manufacturing, IA is now a critical component across various business functions. Combining artificial intelligence (AI), business process management (BPM), and robotic process automation (RPA), IA optimizes business processes by mimicking human cognitive abilities, handling unstructured data, learning from patterns, making predictions, and executing tasks with minimal human intervention. This article explores the top seven IA technologies driving corporate transformation.
The Importance of Intelligent Automation
Intelligent automation is essential for modern enterprises as it enhances efficiency, accuracy, customer experience, adaptability, and cost savings. Here are some key reasons why IA is vital:
- Increased Efficiency and Productivity: IA automates repetitive and time-consuming tasks, enabling employees to focus on strategic and value-added activities. Streamlined processes and fewer manual interventions lead to improved operational efficiency and productivity.
- Improved Accuracy and Quality: By following predefined rules and standards, IA reduces human error, ensuring consistent and accurate task execution. This minimizes data entry mistakes and process deviations, improving data quality and reducing rework.
- Enhanced Customer Experience: IA optimizes customer-facing processes, ensuring faster response times, reduced wait times, and consistent, personalized interactions. This leads to increased customer satisfaction and loyalty.
- Agility and Adaptability: IA’s cognitive capabilities allow systems to adapt to changing circumstances and handle complex tasks. These systems can learn from data patterns, make intelligent decisions, and quickly respond to market changes, customer demands, and regulatory requirements.
- Cost Savings: Automating manual and repetitive tasks reduces labor costs and improves operational efficiency. IA minimizes the need for additional workforce and decreases human-related errors, leading to significant cost savings over time.
- Data Insights and Analytics: IA leverages AI and machine learning algorithms to analyze large volumes of data, extract valuable insights, identify trends, and provide data-driven recommendations for process optimization and decision-making.
Seven Leading Intelligent Automation Technologies
1. Structured Data Interaction (SDI)
SDI involves the integration of well-structured information through traditional systems such as relational databases (RDBMS), data transformation tools, application programming interfaces (APIs), and web services. This technology ensures seamless data exchange and integration across different systems.
2. Robotic Process Automation (RPA)
RPA automates standardized, rule-based tasks using scripts and other methods to support efficient business processes. It is ideal for tasks that are too expensive or inefficient for humans, evolving from isolated automation installations to comprehensive, enterprise-class digital automation solutions.
3. Machine Learning (ML)
ML systems learn by handling variations not anticipated upfront, training on data to make predictions or classifications. For example, ML can map a vendor name to its ID on an invoice, even if the name appears in different forms.
4. Natural Language Processing (NLP)
NLP uses statistical methods and algorithms to analyze text and unstructured information, understanding meaning, sentiment, and intent. In customer service, NLP analyzes support tickets to determine urgency and priority based on the customer’s emotions and frustrations.
5. Natural Language Generation (NLG)
NLG creates text from structured information, such as fields and numerals, to generate reports and insights. For example, NLG can generate sections of financial analysis reports based on a company’s performance data.
6. Chatbots and Virtual Agents
These systems interpret voice/text in free form with predefined answers, continually learning and building vocabulary to improve their responses. In customer service, chatbots can answer queries and provide support, enhancing the customer experience.
7. AI-Decision Systems
AI-decision systems use a range of technologies, algorithms, and models to solve complex decision-making issues. These systems, powered by deep learning and cognitive abilities, recognize patterns and apply statistical models to make informed decisions, such as predicting product demand based on weather forecasts.
Trends Shaping Intelligent Automation in 2024
As intelligent automation continues to evolve, several key trends are shaping its trajectory. These trends highlight the increasing sophistication, integration, and impact of IA technologies across various industries.
1. Deepening AI, RPA, and BPM Convergence
A significant trend is the convergence of artificial intelligence (AI), robotic process automation (RPA), and business process management (BPM) into unified platforms. According to new data from Forrester, 48 percent of organizations plan to integrate RPA and BPM into a single IA platform. This integration enables bots to perform more complex tasks, make data-driven decisions, and handle unstructured data. By combining AI with RPA, enterprises can move beyond simple rule-based automation to implement cognitive abilities, predictive modeling, and intelligent decision-making. This convergence allows businesses to optimize processes more effectively and enhance their overall operational efficiency.
2. Expansion into Non-Traditional Sectors
Intelligent automation is rapidly expanding into sectors that have traditionally relied on manual processes. Industries such as healthcare, legal, and education, which have been slow to adopt automation, are now embracing IA technologies to streamline operations. For example, in healthcare, many routine administrative tasks are still performed manually. Legislative changes and technological advancements are driving these industries to adopt intelligent process automation. This expansion allows businesses in these sectors to improve efficiency, reduce costs, and enhance service delivery.
3. Standardized and Ethical Automation Practices
As IA adoption grows, organizations are placing greater emphasis on governance, standardization, and ethical practices. Establishing RPA Centers of Excellence (CoEs) is becoming more common to manage and optimize automation programs effectively. These CoEs ensure consistency, security, and compliance across automation initiatives. Additionally, sustainability and environmental, social, and governance (ESG) reporting requirements are driving the need for ethical IA practices. Active digital workers minimize resource consumption, optimize business processes, and support data governance, promoting sustainability and ethical standards.
4. Internet of Things (IoT) Integration
The integration of the Internet of Things (IoT) with intelligent automation is creating a network of interconnected devices that communicate and share data in real-time. IoT enhances connectivity, data-driven decision-making, and remote management capabilities. By integrating IoT with IA, organizations can develop more streamlined and intelligent automated processes. For example, real-time sensor data from IoT devices can enable manufacturers and warehouse operators to make instant, data-driven decisions, improving operational efficiency and responsiveness.
5. Advanced NLP Technologies
Natural language processing (NLP) technologies are advancing, enabling bots to interpret and process human language more effectively. When combined with automation methods like RPA, NLP-powered bots can communicate with users using natural language, understand inquiries, provide support, and complete tasks based on user inputs. For instance, NLP can be used to monitor user feedback across multiple channels, perform sentiment analysis to gauge customer sentiment, and generate reports based on customer feedback. These capabilities improve automation by allowing bots to handle unstructured data, categorize information, and provide tailored customer support through chatbots or virtual assistants.
6. Augmented Intelligence
Augmented intelligence is an emerging trend where IA technologies enhance human decision-making rather than replacing it. This approach is particularly valuable for high-touch customer service needs that require human intuition and empathy. Augmented intelligence enables data scientists and other professionals to manage large amounts of structured and unstructured data while providing customers with personalized experiences that pure AI cannot always deliver. In this symbiotic relationship, humans make the final decisions while machines handle the data required for informed decision-making.
7. Hyperautomation
Hyperautomation goes beyond traditional RPA by using a wide range of automation tools and technologies to rapidly transform entire business processes. Hyperautomation aims to automate as many business and IT activities as possible, resulting in improved workflows, productivity, and decision-making. It involves not only automating workflows and process steps but also restructuring work to allow individuals to focus on more creative and strategic tasks. By leveraging technologies like AI, machine learning, and advanced analytics, hyperautomation enables organizations to achieve higher levels of efficiency and agility.