Before the invention of photography, there was only one way to copy classical paintings. Replicating the artworks manually! Paintings were often reproduced by the artists himself or by other artists in the same workshop, either by painting them or by drawing or engraving. An engraving was created for more famous paintings and was then used multiple times to print the outline of the original painting. As a result, the paintings created from the print can be easily identified because they are horizontally flipped and have different colors compared to the original. Despite efforts, there are visual differences between the authentic and the replica, even if the same medium had been used.
One of the jobs of the art historians is to break down these visual differences, to study the human positions used by different artists, and to glimpse the forces that influenced them. They examine the content, style, and genre of the artwork and look for connections, making it a complicated business. But a new algorithm reveals a web of artistic links in search of people in several paintings that are similar.
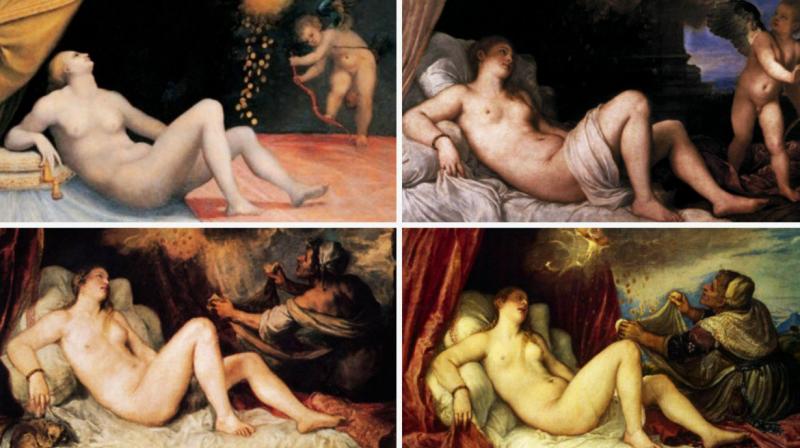
Thanks to Tomas Jenicek and Ondrej Chum at the Czech Technical University in Prague. A machine vision system can scan a painting, analyze the poses of human subjects in fine art paintings and search for other paintings throughout history that contain people depicted in the same poses.
The method works on the use of a vast database of works of art collected from the world’s top museums and galleries, including those found online. The Web Gallery of Art online database contains 37,000 images.
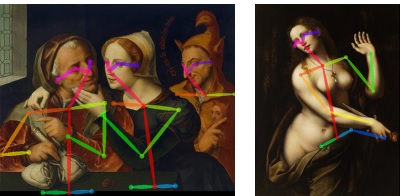
The technique consists of several steps — pose retrieval, fast pose matching, geometric verification, and spatial verification. In pose retrieval, a trained pose detector is used to provide human poses in the image and the detected key points are visualized in a different color. A quick and scalable method is used to identify potential matches. The poses in the images are compared independently, so the relationship between the individual poses is not considered in the first step. Based on the rough geometric alignment of the individual figures, the fast matching step generates a shortlist of potentially similar images, along with the tentative individual pose matches. When potential matches are identified, geometric validation is performed to filter out images for which poses can not be aligned.
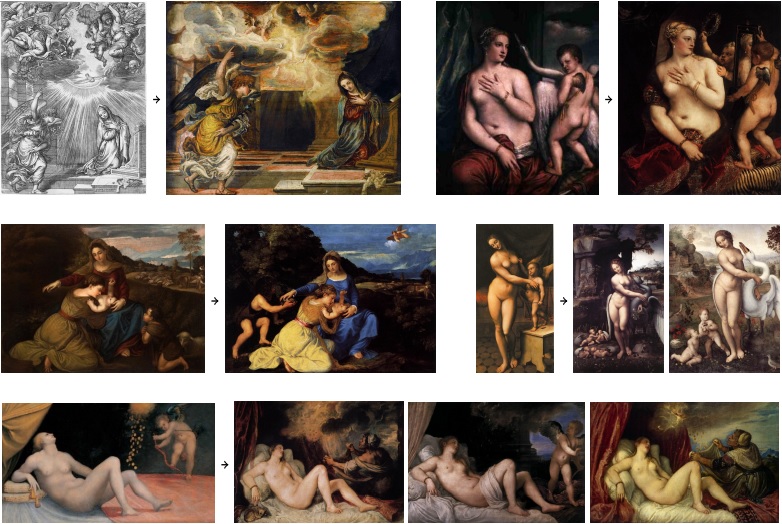
The technique sheds light on previously unknown connections between artists. Historians can use the tool to derive links between different artists and their works. With increasing numbers of galleries and museums choosing to digitize their collections, uncovering relationships between paintings and artists allows art databases to be interconnected without human intervention. It is invaluable for the field of art history to track the theme of inspiration spread throughout time and space as it can provide a survey of a theme’s popularity and evolution as it covers.